I recently posted a working paper where we argue that appointments can substitute for financial commitment devices. I’m pretty proud of this paper: it uses a meticulously-designed experiment to show the key result, and the empirical work is very careful and was all pre-specified. We apply the latest and best practices in selecting controls and adjusting for multiple hypothesis testing. Our results are very clear, and we tell a clear story that teaches us something very important about self-control problems in healthcare. Appointments help in part because they are social commitment devices, and—because there are no financial stakes—they don’t have the problem of people losing money when they don’t follow through. The paper also strongly suggests that appointments are a useful tool at encouraging people to utilize preventive healthcare—they increase the HIV testing rate by over 100%.
That’s pretty promising! Maybe we should try appointments as a way to encourage people to get vaccinated for covid, too? Well, maybe not. A new NBER working paper tries something similar for covid vaccinations in the US. Not only does texting people a link to an easy-to-use appointment website not work, neither does anything else that they try, including just paying people $50 to get vaccinated.
Different people, different treatment effects
Why don’t appointments increase covid vaccinations when they worked for HIV testing? The most likely story is that this is a different group of people and their treatment effects are different. I don’t just mean that one set is in Contra Costa County and the other one is in the city of Zomba, although that probably matters. I mean that the Chang et al. study specifically targets the vaccine hesitant, whereas men in our study mostly wanted to get tested for HIV: 92 percent of our sample had previously been tested for HIV at least once. In other words, if you found testing-hesitant men in urban southern Malawi, these behavioral nudges probably wouldn’t help encourage them to get an HIV test either. That makes sense if you think about it: we show that our intervention helps people overcome procrastination and other self-control problems. These are fundamentally problems of people wanting to get tested but not managing to get around to it. The vaccine-hesitant aren’t procrastinating; by and large they just don’t want to get a shot. Indeed, other research confirms that appointments do increase HIV testing rates—just as this explanation would predict.
This is all to say that the treatment effects are heterogeneous: the treatment affects each person—or each observation in your dataset—differently. This is an issue that we can deal with. Our appointments study documents exactly the kind of heterogeneity that the theory above would predict. The treatment effects for appointments are concentrated overwhelmingly among people who want to enroll in a financial commitment device to help ensure they go in for an HIV test. Thus we could forecast that people who don’t want a covid shot at all definitely won’t have their behavior changed much by an appointment.
But trying to analyze this is very rare, which is a disaster for social science research. Good empirical social science almost always focuses on estimating a causal relationship: what is β in Y = α + βX + ϵ? But these relationships are all over the place: there is no underlying β to be estimated! Let’s ignore nonlinearity for a second, and say we are happy with the best linear approximation to the underlying function. The right answer here still potentially differs for every person, and at every point in time.* Your estimate is just some weighted average of a bunch of unit-specific βs, even if you avoid randomized experiments and run some other causal inference approach on the entire population.
This isn’t a new insight: the Nobel prize was just given out in part for showing that an IV identifies a local average treatment effect for some slice of the population. Other non-experimental methods won’t rescue us either: identification is always coming from some small subset of the data. The Great Difference-in-Differences Reckoning is driven, at its core, by the realization that DiDs are identified off of specific comparisons between units, and each unit’s treatment effect can be different. Matching estimators usually don’t yield consistent estimates of causal effects, but when they do it’s because we are exploiting idiosyncrasies in treatment assignment for a small number of people. Non-quantitative methods are in an even worse spot. I am a fan of the idea that qualitative data can be used to understand the mechanisms behind treatment effects—but along with person-specific treatment effects, we need to try to capture person-specific mechanisms that might change over time.
Nothing scales
Treatment effect heterogeneity also helps explain why the development literature is littered with failed attempts to scale interventions up or run them in different contexts. Growth mindset did nothing when scaled up in Argentina. Running the “Jamaican Model” of home visits to promote child development at large scale yields far smaller effects than the original study. The list goes on and on; to a first approximation, nothing we try in development scales.
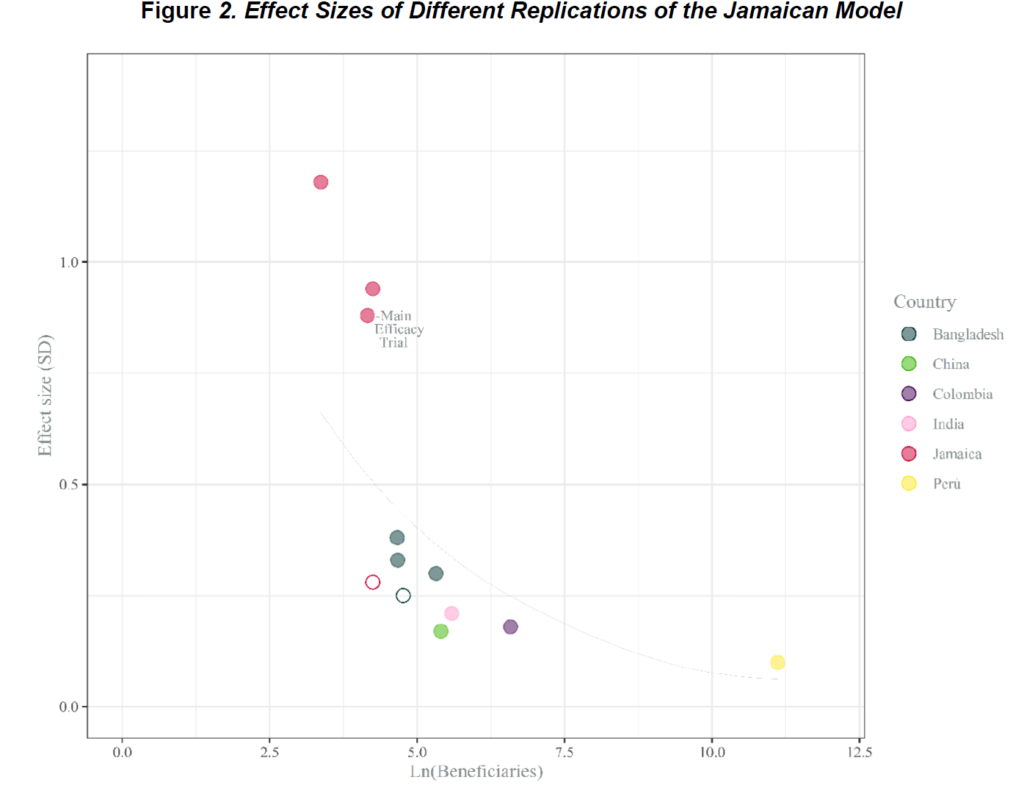
Why not? Scaling up a program requires running it on new people who may have different treatment effects. And the finding, again and again, is that this is really hard to do well. Take the “Sugar Daddies” HIV-prevention intervention, which worked in Kenya, for example. It was much less effective in Botswana, a context where HIV treatment is more accessible and sugar daddies come from different age ranges.** Treatment effects may also vary within person over time: scaling up the “No Lean Season” intervention involved doing it again later on, and one theory for why it didn’t work is that the year they tried it again was marked by extreme floods. Note that this is a very different challenge from the “replication crisis” that has most famously plagued social psychology. The average treatment effect of appointments in our study matches the one in the other study I mentioned above, and the original study that motivated No Lean Season literally contains a second RCT that, in part, replicates the main result.
I also doubt that this is about some intrinsic problem with scaling things up. The motivation for our appointments intervention was that, anecdotally, appointments work at huge scale in the developed world to do things like get people to go to the dentist. I’m confident that if we just ran the same intervention on more people who were procrastinating about getting HIV tests, we could achieve similar results. However, we rarely actually run the original intervention at larger scale. Instead, the tendency is to water it down, which can make things significantly less effective. Case in point: replicating an effective education intervention in Uganda in more schools yielded virtually-identical results, whereas a modified program that tried to simulate how policymakers would reduce costs was substantially worse. That’s the theory that Evidence Action favors for why No Lean Season didn’t work at scale—they think the implementation changed in important ways.
What do we do about this?
I see two ways forward. First, we need a better understanding of how to get policymakers to actually implement interventions that work. There is some exciting new work on this front in a recent issue of the AER, but this seems like very low-hanging fruit to me. Time and again, we have real trouble just replicating actual treatments that work—instead, the scaled-up version almost always is watered down.
Second, every study should report estimates of how much treatment effects vary, and try to link that variation to a model of human behavior. There is a robust econometric literature on treatment effect heterogeneity, but actually looking at this in applied work is very rare. Let’s take education as an example. I just put out another new working paper with a different set of coauthors called “Some Children Left Behind”. We look at how much the effects of an education program vary across kids. The nonparametric Frechet-Hoffding lower bounds on treatment effect variation are massive; treatment effects vary from no gain at all to a 3-SD increase in test scores. But as far as I know nobody’s even looked at that for other education programs. Across eight systematic reviews of developing-country education RCTs (covering hundreds of studies), we found just four mentions of variation in treatment effects, and all of them used the “interact treatment with X” approach. That’s unlikely to pick up much: we find that cutting-edge ML techniques can explain less than 10 percent of the treatment effect heterogeneity in our data using our available Xs. The real challenge here is to link the variation in treatment effects to our models of the world, which means we are going to need to collect far better Xs.
This latter point means social scientists have a lot of work ahead of us. None of the techniques we use to look at treatment effect variation currently work for non-experimental causal inference techniques. Given how crucial variation in treatment effects is, this seems like fertile ground for applied econometricians. Moreover, almost all of our studies are underpowered for understanding heterogeneous treatment effects, and in many cases we aren’t currently collecting the kinds of baseline data we would need to really understand the heterogeneity—remember, ML didn’t find much in our education paper. That means that the real goal here is quite elusive: how do we predict which things will replicate out-of-sample and which won’t? To get this right we need new methods, more and better data, and a renewed focus on how the world really works.
Many projects can be scaled up, if they are set up with that aim and are based on building systemic capacity, such as within a government office. The outcome has to be generally recognized as desirable, unlike a vaccination that has risks and time-limited potential benefit. Your focus on quantitative constructs wastes a lot of time and effort that could be avoided with a better understanding of how people think and act. When I read of the failings of these trials, I find the outcomes so predictable, if the effort had been thought through. But then, if that happened, most trials would not be conducted.
Your focus is on the perfection of your method instead of greater understanding of the issue.
Nice work. As a person who works with policy-makers and philanthropic donors on trying to understand, use & apply decent research about the effectiveness of various interventions, sometimes in order to figure out what to scale… it would help A LOT if academic articles:
1) said how much an intervention cost! It’s amazing that they don’t – even econ journals (economists think about money, right?) So *of course* there’s a good chance that interventions get watered down when people are thinking about scaling them – b/c they might sound expensive, and the paper has no data about whether they actually cost. Bits get lopped off interventions just b/c they sound expensive. (David Evans has an amazing blog post about why economists don’t report costs in their papers. It basically says ‘ooh, it’s all a bit tricky and anyway economists don’t know much about costs’. I’m not an economist, but that seems pretty lame to me…)
2) said what the intervention actually comprised. Out of health came a frustration that so many papers there don’t do this(!) -that is, there’s insufficient detail to know what the intervention actually is and hence to replicate it. Actually, the frustration arose in doing systematic reviews, b/c the meta-researchers couldn’t figure out whether to combine studies or not b/c they can’t tell whether they’re of the same thing. But it obv will thwart a clinician trying to do *the thing* (b/c they can’t tell ‘what thing’).
Hence they created TIDIER – Template for Intervention Description and Replication (TIDieR) checklist and guide. (‘They’ being Prof Tammy Hoffman and co.) It’s basically just who, what, where, when, in what quantity, trained / monitored by whom, using what, etc.
Would be ace if economists (and others) could use that checklist to describe the interventions.
e.g., my org, Giving Evidence, did an evidence & gap map of studies about institutional responses to child abuse*. Loads of the studies just say ‘a six week programme’ but the reader gets no clue as to what was done or by whom in those six weeks. So of course we can’t replicate it accurately.
{www.giving-evidence.com/csa}
This TIDIER framework is a great idea!
On the cost front: it is often pretty hard to work out the marginal cost of an intervention, although I usually do try. In reality a lot of the key variable costs are rolled up with fixed costs, so it’s a lot of painstaking looking at spreadsheets and judgment calls.
Would like to add a third way forward: estimate treatment effects when incentives to do something are watered down when an intervention goes to scale. These incentives (ranging from extrinsic financial incentives through to intrinsic incentives such as an increased sense of importance) have a big impact on how people behave.
I find this to be useful: https://www.bsg.ox.ac.uk/sites/default/files/2018-06/2017-07%20Policy%20memo%20External%20validity%20and%20policy%20adaptation%20%20Williams%20.pdf.
Very well-written and thought-provoking article about the heterogeneity effect. One wonders whether implementation science research should be a mandatory step to attempting to scale any intervention that has been shown to work in the setting of an RCT. It may help tease out those real-world factors that would make a tested intervention fall flat on its face.